A new machine learning tool that assesses surgical risk—dubbed Pythia— helps amplify the expertise of Duke providers in predicting postoperative outcomes for different types of high-risk surgeries. The tool was developed by the Duke Institute for Health Innovation.
In this Q&A, Oren N. Gottfried, MD, a neurosurgeon at the Duke Spine Center, discusses the role of predictive analytics in personalized spine surgery and the ways in which Pythia helps providers and patients make better-informed health decisions.
Q: How does Pythia use machine learning to help patients and physicians?
Gottfried: This tool draws patient data directly from the electronic health record so we can have complex, real-time data at the point of care. It can assess a comprehensive set of data points from more than 100,000 patients treated at Duke without any effort on the part of the surgeon. As a clinician, I report to my patients about the possible outcomes of their spine surgery based on my experience, the available surgical literature, and the complex and multiple iterations the system runs for us, which in turn makes the patient more informed when they’re making clinical decisions.
Q: How does Duke’s risk calculator improve on the National Surgical Quality Improvement Program (NSQIP) to predict patient outcomes for surgery?
Gottfried: NSQIP’s predictive model is based on hundreds of thousands of patients throughout the country and enables a surgeon to assess statistically the predicted expected outcomes. However, that program requires manual data entry and does not include all of the details or codes associated with the surgery. Although NSQIP is a great tool, Pythia is an even better predictive tool because it is built and based specifically on our local patient population.
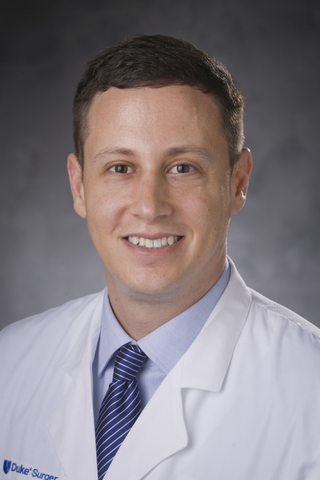
Q: How does Pythia fit into a patient’s assessment for spine surgery at Duke?
Gottfried: The goal with Pythia is to use machine learning to identify spine patients who are at highest risk for postoperative complications. Again, the best predictive tool is based on your own patient population, and we have started using Pythia for spine and it is used for other surgical specialties. It is great that Pythia uses real-time data—without a clinician needing to enter it—that gives us predictions of how that patient would do compared to their peers with a similar condition or surgery. This type of tool is the future of spine surgery, and Duke is ahead of the curve in terms of how we look at and value these data.
We use Pythia’s predictive analytics in two main ways:
- To help us understand where someone’s health could be optimized before surgery, to help select patients for preoperative geriatric or anesthesia consultation and treatment, and to assess if the patient is better suited for a less invasive surgery or nonoperative treatment.
- As part of our weekly multidisciplinary indications conference for spine, where we decide which cases would benefit from surgery or other treatments. The tool is used for other conferences including with our quality group.
By using this tool to assess preoperative optimization and postoperative outcomes, we’ve seen a very nice trend in improving our rates of readmissions and length of stay and other outcomes within our health system. It's because of these predictive analytic tools and modeling that we're able to better understand with the highest accuracy the severity of a patients’ illness.
Q: What other metrics do you hope this machine learning tool can assess in the future?
Gottfried: In the future, I’d like to see if we could predict patient satisfaction with surgery so that it’s not just an objective outcome. We’re working toward improving the patient’s overall life experience in addition to improving their functionality and other more objective aspects. We're also looking deeply into other metrics to improve, including return-to-work times, reduced time off from work, and quality of life, which can make a big difference in patient satisfaction rates.